Rocco Van Schalkwyk 15/10/2022
Like many aspects around the Xzistor Concept brain model, the discussion around how it implements a speech and language capability will require a mental journey many current linguists and AI researchers are not ready for yet. This is because it involves machine emotions – a concept not many are ready to accept as a real or implementable at the time of publishing this post.
A few Xzistor LAB collaborators have worked on their own theoretical approaches towards brain modelling which have provided them with an understanding of machine emotions, and specifically how machine emotions are implemented within the Xzistor Concept. These individuals now have the basic understanding to appreciate the scientific basis of how machine emotions can be designed and implemented in Xzistor artificial intelligent agents. With machine emotions as the basis, it becomes easier to explain how a speech and language facility can be achieved in artificial agents using the Xzistor Concept cognitive architecture.
Quick Refresher on Machine Emotions
According to the Xzistor Concept there is only information in the brain. To make a brain state ‘bad’, the brain state must be turned into an ‘avoidance’ state – because the robot will learn to perform effector actions to avoid it. To make it ‘good’ the brain state must be turned into a ‘approach’ state – because the robot will learn to perform effector actions to pursue it. By using sensory-type states and turning these into ‘avoidance’ or ‘approach’ states that can subjectively be ‘felt’, these sensory-type states can be given motivational value – ‘avoid’ (bad) or ‘approach’ (good) – as wells as motivational saliency (strength). The agent will constantly be aware of these sensory-type ‘feeling’ states as they will be subjectively felt – and we will refer to them as negative (-) or positive (+) machine emotions.
Most of the agent’s behaviors will originate from learning how to evade ‘avoidance’ states and achieve ‘approach’ states. This will happen through special reinforcement learning events called Satiation Events as part of operant learning. Operant learning happens when moving out of an ‘avoidance’ state and into an ‘approach’ state is rewarded by the brain by storing the effector actions that led to securing access to the reward source as an association. In humans, the subjective feelings experienced during the reward stage are often termed ‘relaxing’ or ‘pleasurable’ because what the brain has been trained to avoid (the bad state) is being reduced.
The manner in which the Xzistor Concept defines machine emotions cannot be fully understood without introducing two important theoretical constructs, namely Body UTRs and Brain UTRs. A basic understanding of these two functional mechanisms will aid in understanding that there are basically two types of machine emotions that can be generated in the brain of the Xzistor artificial agent.
Body Urgency To Restore mechanisms (or just Body UTRs) are simple homeostasis-type control loops for avoiding e.g. thirst, hunger, cold, pain, fatigue, etc. Each of these has its own dedicated centre(s) in the biological brain where the ‘avoidance’ and ‘approach’ brain states are generated based on the measured parameters on which the homeostasis mechanisms are based (utility parameters like hydration level, nutrition level, tactile pressure on the skin, etc.). These states are all subjectively experienced in the brain and constitute the first type of emotion that we can create machine equivalents of.
But these emotions, like experiencing intense thirst, hunger or pain or reciprocally drinking (thirst quenching), eating or escape for pain cannot be regenerated when recalled from memory in future. For instance we do not suddenly experience the bad feeling of thirst again when we think about it. Memories that make us feel bad when we recall them make use of another type of emotion. They emanate from a stress state that is parasitically generated at the same time a subjective state like thirst, hunger or pain is generated. In the human brain this is the Sympathetic Nervous System (SNS) that is automatically activated at the same time as states like thirst, hunger, pain. etc. These turn into a subjective feeling when they affect the vagal nerve (digestive tract) through e.g. adrenaline/noradrenaline/cortisol release. The sensory state in the gut is projected by sensory neurons through the brainstem to the cortical areas in the brain where a subjective sensory state is created. The SNS cause this avoidance state in the somatosensory area, and it will increase (in activity) and then decrease (in activity) when the SNS state is inhibited by the Parasympathetic Nervous System (PNS). These are the ‘avoidance’(stress) and ‘approach’(relax) states that indeed can be remembered, and these emotions or ‘gut feelings’ will be recalled as emotions when we see or think about the conditions that caused them in the first place. As the Body UTR mechanisms get triggered, they will always create their own unique ‘avoidance’ and ‘approach’ states (e.g. feeling thirsty, hungry, etc.) as well these automatic SNS (stress) and PNS (relax) states that will be contributed to by all the different Body UTRs active at the time. The SNS (stress) and PNS (relax) states are the effect of all the active Body UTRs working on the same system and will result in a single collective ‘gut sensation’ either good (agent must pursue) or bad (agent must avoid). Whilst subjective Body UTR sensations like thirst, hunger and pain cannot be regenerated by recalling memories, the SNS (stress) and PNS (relax) states will be stored as part of associations and will be re-evoked (regenerated) when the human or artificial agent sees or thinks about the conditions that triggered them.
When we regenerate these SNS (stress) and PNS (relax) states because we recognize an object or think about an event to which they were linked, they effectively constitute ‘emotions from memory’ and will again trigger adrenaline/noradrenaline/cortisol (SNS) or inhibitors (PNS) for these. We will call these mechanisms Brain UTRs. Just like Body UTRs these good or bad emotions regenerated from memory could be strong and add to or oppose what the brain is already experiencing. Since they only work on the vagal nerve ‘gut feeling’ they will compete with other emotions that are affecting the SNS and PNS states as a result of activity by Body UTR mechanisms. So the different machine emotions that can be created are:
- Unique ‘subjective’ emotional states generated by active Body UTRs like thirst, hunger and pain.
- The correlating SNS/PNS emotional states as a result of those same active Body UTRs.
- The SNS/PNS emotional states as a result of what is sensed or thought about from memory.
Whilst all the unique Body UTRs will separately be experienced as recognizable subjective states like thirst, hunger, pain, fatigue, etc. – the effect these mechanisms have on the SNS/PNS will just cause a single resultant state (emotion) experienced in the gut through the vagal nerve ascending pathways to the cortex.
Although explained here at the hand of the biological brain, the Xzistor Concept will build simple equivalent systems that achieve the machine equivalent of these mechanisms.
For more on Xzistor Concept machine emotions please refer to this blog post on the Xzistor LAB website: https://www.xzistor.com/machine-emotions/
Or the author’s original free short book on machine emotions:
Understanding Emotions: For designers of Humanoid Robots
Early Xzistor Embodiments – Simmy Simulation
As the inventor of the Xzistor Concept brain model, I have not in any great detail implemented language learning in either my Simmy simulation or Troopy physical robot (please see www.xzistor.com). I have however spent quite a bit of time and effort on doing some early preparations for more comprehensive tests and demonstration using the Xzistor cognitive architecture.
Going back 20 years, I added into Simmy’s learning confine a ‘speaker’ which was able to send an audio message ‘Yes!’ sound message (green indication) and a message ‘No!’ sound message (red indication).
Added: I just managed to find the legacy video clip below of Simmy hearing the ‘No!’ command over the speaker. The emotional effect is not that clear because the little agent is already very distressed. This is because its stomach (backpack with purple fuel) is empty. But even so one can see how the corners of its mouth dips down even further when it hears the ‘No!’ command and also see the slight spike in brown bars on its Emotion Panel. Click on image below to start short video:
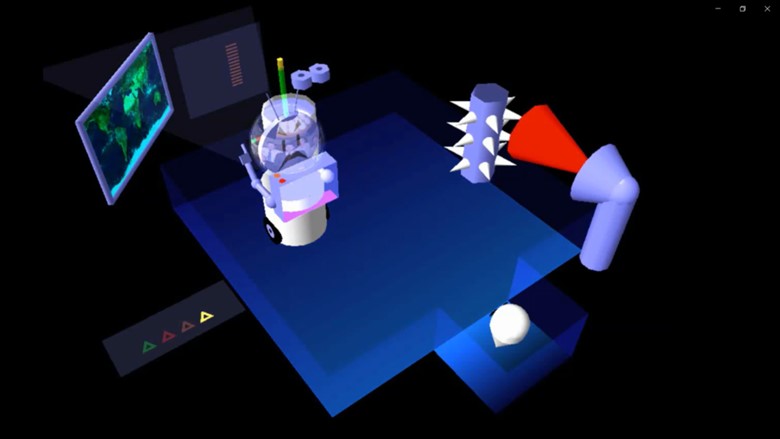
With the push of the N-key on the keyboard, I could ‘activate’ the speaker and the agent will hear the ‘No!’ word and turn this into a brain state or representation. This demonstrated that by using the word ‘No!’ while an avoidance state is being experienced, the representation of this sound state will become part of the association that is created at that moment, and then hearing this sound again in future will re-evoke a negative emotion (SNS (stress) activation state as a -%) e.g. when Simmy hears the word ‘No!’ again even in other situations or contexts, it will feel a sense of avoidance (SNS activation) created by the SNS/PNS emotion mechanism.
Similarly, the word ‘Yes!’ would become part of a positive approach (pursual) state association and become something that can trigger positive PNS emotions as a +% (based on inhibition of the SNS) when the association is recalled.
This is a good example of a Brain UTR. This Brain UTR will generate SNS Deprivation (-%) when the word ‘No!’ is heard and the agent will want to do something to ‘avoid’ this negative emotion. It will have to learn what it is that it needs to do and in different contexts as these actions could differ. If it is moving up towards the edge of the swimming pool and it hears ‘No!’, and it then reverses and hears ‘Yes!’ (which will generate a positive emotion PNS state), it will experience a Satiation Event and learn that the correct action to avoid/diminish the Deprivation state (SNS) from the word ‘No!’, when close to the swimming pool, is to reverse away.
This simple process opens the door to a very powerful educational process.
Originally, everything an Xzistor agent learns is through operant learning. The effector motions leading to grasping and eating an apple (simulated of course) will trigger a Satiation Event that causes learning. Similarly, if we just regard the speaker (voice) of the agent to be another effector, it can produce pressure waves sounding like ‘Give me the apple!’. This could also secure access to the apple as a reward source and broadcasting this sound pattern will become reinforced as an effector action (compulsion) when next the agent is hungry and close to the apple. In fact, this will become the effector action of choice because it entails less effort (cost) to secure the reward source.
Whereas with a human baby a long process of babbling simple sounds and words need to precede the point where mimicking the mother’s words / sentences becomes a source of Satiation in itself, and learning can occur, we can implement a much more expedited process with Xzistor agents.
We can give the Xzistor agent a mimic reflex.
Preceding handing over the apple, we can say ‘Give me the apple!’
The agent will then repeat: ‘Give me the apple!’ and on that moment we hand the agent the apple, it eats it and goes into Satiation meaning a Satiation Event takes place and it remembers the hand motions as well as the sounds it projected through the speaker that contributed to the successful eating event.
While the robot eats, we say ‘Good robot!’
And the robot repeats ‘Good robot!’
Important: The words ‘Good robot!’ is now locked into memory with a strong Satiation association and in future when the agent hears those words, whether hungry or not, the PNS (relax) emotion will be triggered – creating a positive emotion (we might even see a little smile on the agents’ face).
Because the words ‘Good robot!’ now triggers PNS Satiation – it can be used as an operant learning reward state. For anything the robot now does, we can say ‘Good robot!’ and the robot will remember those actions as a Satiation Event with operant learning. It will become eager to please (because it will find Satiation).
The robot can now be made to do things (and say things) in return for emotional reward.
If we now put an image of a pineapple in front of the robot and say: ‘Pineapple!’ the robot will mimic the word and also say ‘Pineapple!’ and if we then say ‘Good robot!’, a Satiation Event will occur that will lock the image of the pineapple with the words ‘Pineapple’ spoken by the robot into the robots association database.
In this way we can teach the robot to start to link images to words and slowly its vocabulary will start to grow.
The above process will not yet involve any understanding of grammar or sentence structures.
As the process continues the robot will learn that a bad sentence e.g. ‘Give him apple!’ will not lead to reward and only the correct sentence ‘Give me apple!’.
Later the robot will learn that just the word ‘Give!’ can be used to get handed an object from the tutor. This will happen because of the Xzistor model ‘context’ generator (and directed Threading). This algorithm ‘try’ random words from sentences in situations where agents have not been able to learn before. The model chooses these, and other actions, from closely related associations, as part of ‘intelligent guessing’, to solve problems i.e. to evade ‘avoidance’ states or achieve ‘approach (pursual)’ states. This is informed by similarity in sensory states, cues from the environment or other associative artifacts based on emotion value (+ or -) and salience. If a guessed action or word leads to Satiation, a Satiation Event will occur, and the reward will mean the action (or word) becomes the chosen action to perform in future to solve the newly encountered problem. This can solve a completely novel problem in unfamiliar environments – and is basically how humans also solve novel problems in unknown domains (there is often a bit of guesswork involved).
An example to illustrate this logic mechanism could be where the robot moves towards an open fire and the tutor shouts ‘No!’. The robot has never encountered this situation or seen fire before. But the ‘context’ algorithm will collect any associations that could relate to what is being seen, heard, felt etc. through a process called ‘directed Threading’. This subset of associations (some perhaps only vaguely related or sharing a weak link / attribute) will now be presented to the robot brain in order of preference based on similarity, emotional value and emotional saliency. The robot brain will be encouraged to ‘try’ the motion commands stored as part of these associations. Let’s assume one of the ‘context’ associations presented to the robot brain is the one where the robot has learnt to reverse away from the swimming pool. The robot activates this motion command set by recalling the stored association motions and reverses away. Suddenly the robot hears the tutor say ‘Yes!’. There and then a Satiation Event takes place in the robot brain as the negative emotion associated with ‘No!’ changes to the positive emotion associated with ‘Yes!’. The robot has just learnt through operant leaning that when it gets close to a fire, it must reverse away. This is how the Xzistor agent will use past experience to solve novel problems in new environments. If it has learnt to open a blue cupboard door to find food, it might enter a new room when hungry and see a green cupboard door that looks different but have some common features like doorknobs or keyholes. The ‘context’ mechanisms will now encourage the robot brain to try the actions it performed to open the blue door on the green door. This might lead to the robot finding out how to open the green door all by itself – and in this case without the help of the tutor. Finding the reward will reinforce the actions that were successful.
When it comes to the words spoken by the robot, the grammar in these sentences will only be based on what works and what does not work. And this can be expedited with the tutor saying: ‘Good robot!’ when the correct sentence construction is used. Just like the objects in an optic image will start to get individual meaning as they are singly experienced in different contexts, so words will also get individual meanings. For instance when the robot says ‘No!’ it will learn that the tutor will act helpfully and remove the scary toy, but on another occasion also take away the bad tasting food. ‘No!’ now becomes detached from just one context and starts to serve as an expression (effector action) that can in different situations make avoidance states or objects disappear or be reduced.
The robot will learn that using the wrong sentence construction will delay getting reward sources and could generate disappointing ‘No!’ and ‘Bad robot!’ responses from the tutor (it is important to appreciate that the tutor will become a strong emotional reward object / source in the life of the robot). Question: Is this perhaps the basis of love?
Using a whole sentence to elicit a reward will be no different from executing a sequence of effector actions to get access to a reward. The words become the individual effector actions and they will be subject to reinforcement based on prediction errors (changes in anticipated Satiation) – just like doing the sequence of manual actions to get to a rewards source, placing the words in the correct sequence will lead to success and reinforcement.
Interesting: There is principally no difference between a manual effector action and pronouncing a word – and only executing these in the correct order will ensure access to the reward source.
We should therefore see the Xzistor agent learn to use the correct sentences to achieve goals.
Adding prefixes etc. as per grammar rules will be formed by learning (habit) and only much later when taught about grammar, sentence construction and writing – will any notion of grammar or sentence construction become known to the agent.
Xzistor robots learn just like humans.
Early Xzistor Embodiments – Troopy Physical Robot
The ‘mobile feeder’ below was used to start experimenting with Troopy around the theoretical aspects discussed above. See video on YouTube: https://youtu.be/7H0gUwAYnQo
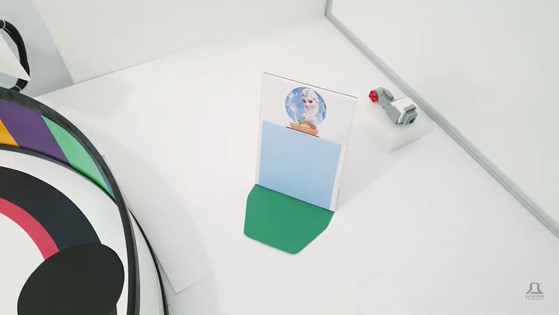
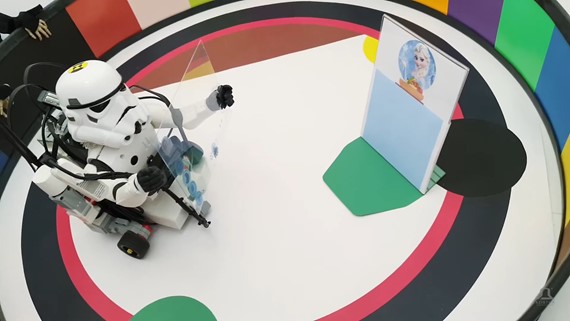
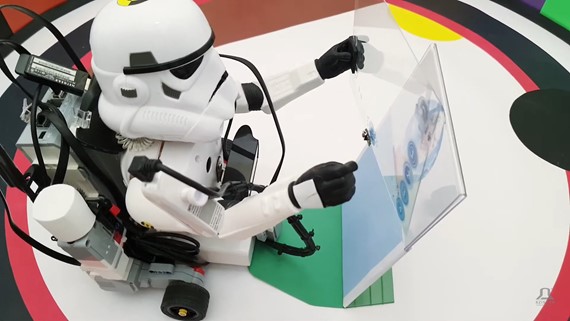
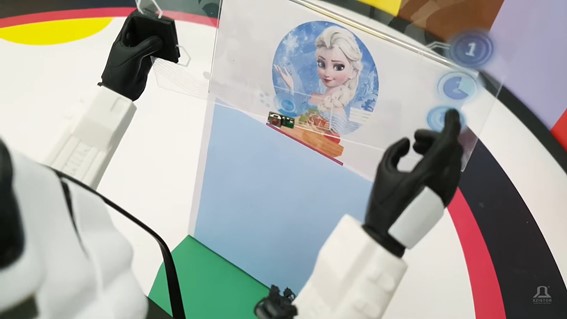
Advanced Speech and Language Facility
After much more learning (years), Xzistor robots will learn that using correct words and word sequences will impress people and ensure clear communication. Then speaking will also become linked to reading and writing, and will become a means to convey complex thoughts – not just short cues to command rewards but sharing abstract thoughts and ideas. But during the early years, language learning will prominently revolve around restoring the basic homeostatic needs generated by Body UTRs and Brain UTRs and this will quickly progress to a strong tendency to try and please the tutor as this will lead to instant ‘emotional’ reward e.g. upon hearing the words: ‘Good robot!’.
A next phase more advanced Xzistor language development experiment should be very interesting as it could be based on what was discussed above. Again, the aim of the Xzistor project is not to see how far we can push the cognitive architecture but rather to systematically build evidence of how it ‘principally’ explains the working of the brain when it comes to an artificial speech and language facility.
Refining this into more complex implementations with higher resolution and with the ability to process large amounts of complex information can be added as a next phase.
iCub Robotic Platform Speech and Language Facility using Xzistor
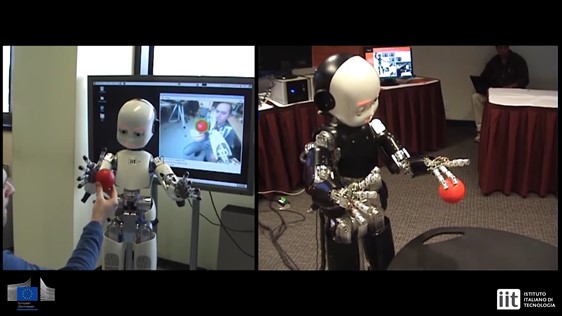
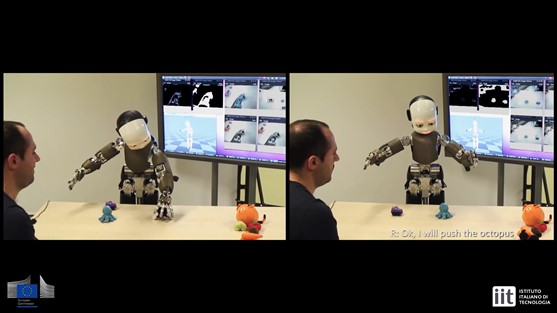
How would a simple iCub robotic platform implementation work using the Xzistor Concept cognitive architecture?
1.) Install the Xzistor cognitive architecture on the iCub platform (the Simmy simulation was written in C++!)
2.) Teach iCub to access apple when hungry.
3.) Teach iCub to say ‘Give apple!’ by mimicking and operant learning.
4.) Teach iCub to associate ‘Good robot!’ with PNS Satiation (+emotion) during eating reward.
5.) 4. above can now act as reward source (Satiation Event) for iCub.
6.) Teach iCub more words by showing image, naming the object in the image, waiting for the robot to mimic, and rewards with: ‘Good robot!’
7.) Gradually implement longer sentences.
8.) Allow the context generator to break up sentences into single words and use them in other contexts e.g. [give] [my][blue][ball][please].
I fundamentally belief there are dedicated innate structures in the biological brain aiding in building an efficient speech facility. But this can be the basis of future studies based on the simple principles provided by the Xzistor Concept. There is a lot that can be explored not just refining the general model, but refining detail around the functionality of speech, writing and reading.
Final Thoughts
For now, I suggest following a modest route towards more complex implementations as overcomplicating a demonstration programme could distract from a basic model that ‘principally’ explains what happens in the brain – a crucial first step. I see the Xzistor Concept as a basic ‘Bohr Atom of the Mind’, a brain model that is now desperately needed after decades of searching for such a model in vain. A good way forward will be to use the speech facility demonstrated by the Xzistor Concept and then build on it – refining aspects to it and adding complexity – all which will be possible and will help elucidate the logic and underlying mechanisms of speech and language. This will hopefully help linguists and AI researchers gain a more complete understanding of how this complex functionality is achieved by the biological brain and how it can be replicated synthetically.